Big Data Analysis Stucks Trust Problem Faces Big Test Forrester Research launched a survey in July 2016 to investigate 2,165 data and analytics decision makers managed by KPMG (KPMG). The report shows that data and analysis tools have been widely used to analyze existing customers (50%) and discover new customers (48%). However, only about 34% of business leaders “very trust†tools to analyze the results of business operations data.
There are signs that investment in data and analytical tools is increasing. Therefore, Bill Nowacki, an expert in data analysis, believes that the degree of trust in the results of the analysis is certainly better. In an interview with the SearchDataManagement organization, Nowacki said the analysis tools needed to be more transparent. In other words, the analysis tool should show management the logic behind the recommendations and show the basis for the analysis tools to give conclusions.
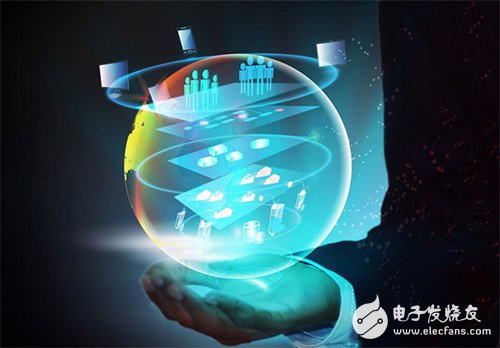
The findings seem to focus on the gaps in data trust. What is the trend of confidence in data quality in the future?
Bill Nowacki said that the core concept of "analysis is essential" is now basically accepted. However, at the same time, our training for management is not enough. The industry should generally take certain measures.
We can see from the statistical chart of the survey report that the overall acceptance of the analysis is shown as a U-shaped trend. Looking back a few years ago, it was really like the "western wild age". Everyone was building various analytical models and buying them quickly without full review. These tools are included in the regular decision making. This state lasts for a long time.
Later, we saw that some decisions were not as accurate and optimal as expected. The true credibility of the model faces great challenges. Now, we are at the bottom of the U-shaped trend, but we are beginning to wake up, and now it is time to look at it with a more serious attitude. We need to consider which data to use, where the data comes from, whether the data is correct enough, and so on.
There are already some predictive models in the industry that are in use, especially in marketing and pricing. People use models for optimization or asset management. We see many similar cases. However, if it involves the company's key business, we must consider it carefully to ensure business stability. We are beginning to see the other side of the U-shaped trend – the uptrend after the trough, but structural rationality, governance, and compliance require more analytical transparency.
Machine learning for predictive analytics does not improve transparency. It seems that it is not easy to open the "black box" to help improve data trust.
If you look back at the development of FICO (Global Forecast Analysis and Decision Management Company), you will find that in the 1980s and 1990s, they have used neural networks to achieve better and highly predictable credit scores. However, what is missing is still the ability to explain.
The regulator will come and tell me, “If you want everyone to believe, you must be able to explain why.†When the “Western Wild Age†model was born, everyone needed better transparency. We need to understand why the engine gives these ideas and understand what these ideas stand for.
If the tools are "black boxes," it's hard to trust them. Therefore, transparency should be considered when making choices during the design phase, so that some trust can be gradually developed.
Today we are seeing a more thoughtful design, we spend time thinking about how we can achieve better results, so that we can make great progress. For example, people check their retail store portfolio and use all signatures or registration information to discover a truly high-quality customer base across all stores. You can get all the real samples, you can set the target price in this group to see if you can get the expected results. Once it is confirmed that the price adjustment is effective in a small customer base, it can be implemented to a larger group one by one. This small step of change is easy to achieve.
Executives have gradually accepted analysis for various businesses, but nothing is more convincing than success stories. We should look at the market thoughtfully, look at the test objectives we set and validate our previous predictions of using big data to market, which can also help increase trust in data analysis tools.
KNL1-63 Residual Current Circuit Breaker
KNL1-63 Moulded Case Circuit Breaker is MCCB , How to select good Molded Case Circuit Breaker suppliers? Korlen electric is your first choice. All moulded Case Circuit Breakers pass the CE.CB.SEMKO.SIRIM etc. Certificates.
Moulded Case Circuit Breaker /MCCB can be used to distribute electric power and protect power equipment against overload and short-current, and can change the circuit and start motor infrequently. The application of Moulded Case Circuit Breaker /MCCB is industrial.
Korlen electric also provide Miniature Circuit Breaker /MCB. Residual Current Circuit Breaker /RCCB. RCBO. Led light and so on .
KNL1-63 Molded Case Circuit Breaker,KNL1-63 China Size Molded Case Circuit Breaker,KNL1-63 Electrical Molded Case Circuit Breaker,KNL1-63 Automatic Molded Case Circuit Breaker
Wenzhou Korlen Electric Appliances Co., Ltd. , https://www.korlen-electric.com